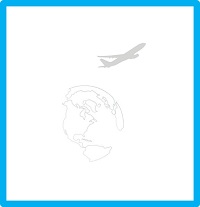
Machine Learning For Optimizing Aircraft Manufacturing Processes
The aerospace industry is extremely competitive, with aircraft manufacturers under constant pressure to produce high-quality products efficiently and cost-effectively. One way companies can improve their operations is by leveraging advanced technologies like machine learning and artificial intelligence. Implementing ML solutions throughout the manufacturing workflow can lead to reduced waste, faster production times, and higher quality standards.
How Machine Learning Enhances Manufacturing
Machine learning algorithms uncover patterns in large datasets to make predictions or recommendations. In manufacturing, ML can be applied to:
- Predict equipment failures and recommend preventative maintenance
- Optimize supply chain logistics
- Improve inventory management
- Enhance quality control with automated inspection
- Personalize workforce training based on individual strengths/weaknesses
With massive amounts of data generated throughout the aircraft assembly process, there is huge potential for ML to pinpoint inefficiencies and variability. Continuous improvement is critical in aerospace, as tiny defects could compromise safety. ML allows manufacturers to identify root causes of issues, take corrective actions, and prevent future occurrences.
Key Applications of ML in Aircraft Manufacturing
While the entire end-to-end aircraft production workflow can benefit from ML, some key applications include:
Predictive Maintenance
Aircraft are made up of millions of parts, involving thousands of manufacturing and assembly steps. All the machinery and equipment used in the production process is prone to wear and tear over time. ML algorithms can analyze sensor data from machines to identify signs of deterioration and predict potential failures before they occur. This prevents unplanned downtime and costly disruptions.
For example, sensor data on vibration, temperature, and pressure can train ML models to flag when equipment needs maintenance. Automated alerts allow issues to be addressed early. ML even identifies optimization opportunities – like adjusting machine operating parameters – to extend equipment lifetime.
Automated Visual Inspection
Traditionally, visual quality checks on aircraft components are performed manually. But computer vision AI is revolutionizing inspection. ML algorithms can review images and videos of parts to identify any scratches, dents, missing pieces, alignment issues, and more. This ensures stringent quality standards are met.
Computer vision can rapidly scan manufactured parts of all sizes and materials – from small fasteners to large fuselage sections. ML models can be customized for different inspection tasks and improve over time as more data is aggregated. Automated optical inspection is extremely precise, objective, and efficient – surpassing human capabilities.
Supply Chain and Inventory Optimization
Aircraft manufacturing supply chains are massive, with thousands of parts needing to be sourced from different locations and meticulously scheduled for just-in-time delivery to assembly plants. ML helps optimize inventories and logistics to minimize costs and delays.
By analyzing supply needs, production schedules, delivery times, weather forecasts, and other data, ML models can improve demand planning, inventory management, and traffic coordination. This ensures smooth material flows to achieve on-time production. Models can also determine optimal warehouse layouts and stock replenishment policies.
Automated Production Scheduling
Detailed scheduling of all manufacturing activities is required to ensure on-time aircraft delivery. This involves optimizing sequences, resource allocations, and material flows. Manual scheduling is extremely complex, leading to inefficient plans. ML scheduling algorithms leverage data like aircraft configurations, production capacity, resource availability, and order backlogs to rapidly generate optimized build schedules.
The AI continuously adapts schedules by incorporating new constraints, workloads, and variability. This level of agility and precision is impossible with manual methods. Automated scheduling minimizes delays and improves productivity.
Anomaly Detection
Aircraft production generates massive volumes of data across the assembly line. Anomaly detection uses ML algorithms to analyze this data and pinpoint patterns that deviate from normal behavior. Abnormal events could indicate emerging defects, equipment malfunctions, cybersecurity breaches, or other issues requiring intervention.
Early anomaly detection allows corrective actions to be taken before problems escalate or quality is impacted. Models are trained on baseline “normal” data first. They then identify outliers in real-time data – whether they be anomalous sensor readings, suspicious network activity, or unexpected product variations. Engineers gain invaluable insights to continually refine processes.
Automated Robotic Assembly
Aircraft manufacturing involves many repetitive, tedious tasks that are ideal for automation. Robotic process automation using AI-driven robots can efficiently perform activities like riveting, fastening, adhesive dispensing, finishing, and painting. Machine learning helps robots adapt to variability and improve precision over time.
Computer vision enables robots to inspect parts to determine optimal gripping points before picking them up. The robots can coordinate with each other to handle tasks in parallel on the production line. Intelligent automation boosts output and minimizes errors.
Generative Design
Generative design ML algorithms allow engineers to define desired aircraft performance requirements and goals. The AI then rapidly generates design concepts optimized to meet the specs. Engineers simply pick the best option.
By iteratively trying millions of combinations and permutations not humanly possible, the ML models identify novel, high-performance designs. This accelerates innovation and reduces development timelines. Augmenting human creativity with AI leads to better products.
Workforce Training
Thousands of technicians, engineers, mechanics, electricians, and other specialists work on aircraft manufacturing. Their skills must continuously improve as technologies evolve. ML algorithms can track individual ability levels and knowledge gaps based on assessments.
The ML systems then deliver personalized, interactive training content precisely tailored to each worker. This targeted approach accelerates skill gain. The algorithms also recommend best team configurations based on members’ strengths. Optimized teams minimize errors and boost productivity.
Implementing ML Solutions
To effectively implement enterprise-wide ML, aircraft manufacturers need:
- Skilled data science teams – ML success requires data experts that can build, test, and deploy models.
- Integrated data infrastructure – All data sources must be aggregated into a unified platform to feed ML models.
- Management buy-in – Leadership must provide resources, oversight, and direction to data science teams.
- Training programs – Workers across functions need to learn new data-driven ways of working.
- Change management – Culture and mindsets must evolve to become more data-oriented.
- Governance frameworks – Ethical oversight of ML is critical, including bias detection.
The benefits of ML must be demonstrated through controlled pilot projects first. With an iterative, test-and-learn approach, use cases can be expanded gradually organization-wide. Patience is key – ML adoption involves cultural transformation alongside technology implementation.
The Future of Machine Learning in Aircraft Manufacturing
ML is already transforming aircraft manufacturing, but Big Data tools will amplify its impact even more in the future. 5G networks, internet of things sensors, and edge computing will enable an explosion of manufacturing data. Cloud platforms will allow infinite storage and computing power for analyzing this data.
With more data, ML algorithms will become even more accurate and new use cases will emerge. In the long run, “digital twin” simulations of entire aircraft factories may optimize every aspect from staffing to floor layouts. AI will work alongside humans to achieve unprecedented levels of efficiency and agility.
The factories of the future will blend physical and virtual worlds, coordinating humans, robots, and AI in a seamless flow of information and action. Aircraft manufacturers that leverage these technological capabilities will lead the industry. With the accelerating pace of ML innovation, the opportunities are sky-high.
Frequently Asked Questions
Here are some common FAQs about using machine learning to optimize aircraft manufacturing:
How can ML improve quality control?
ML algorithms can analyze visual data from parts and automatically identify any defects. This automates inspection and boosts quality. Models also detect anomalies in manufacturing data to pinpoint issues.
What manufacturing processes benefit the most from ML?
Predictive maintenance, supply chain management, and scheduling see the biggest ML improvements. Automated visual inspection and robotic assembly also improve with ML.
How does ML reduce manufacturing costs?
ML optimizes uptime, inventory, logistics and quality. This reduces waste, saves time, and prevents costly errors. Efficient algorithms also cut computing costs.
What are the challenges of implementing ML in manufacturing?
Key challenges include data integration, finding data science talent, change management, and demonstrating quick ROI from projects. Robust data governance is also critical.
How can ML algorithms adapt to new aircraft designs?
With a flexible ML architecture, models can be retrained with data from new parts and products to update predictions and recommendations. Transfer learning also helps apply learnings across design changes.
Can ML reveal insights humans would miss?
Absolutely. By processing millions of data points, ML uncovers hidden patterns and non-intuitive connections that humans cannot discern through manual analysis.
How can manufacturers reskill workers to use ML tools?
Use ML itself! Adaptive learning algorithms identify knowledge gaps and deliver personalized training to upskill each worker. Gamification helps make learning engaging.
Will ML replace or augment manufacturing jobs?
ML will automate repetitive and dangerous jobs. But it will augment skilled workers, allowing them to focus on higher-value and creative tasks. New roles like data scientists will emerge.
How can manufacturers ensure ML ethical compliance?
Rigorous testing for biases and fairness is essential. Having diverse data science teams also improves model outputs. Ongoing audits coupled with human oversight of all automated ML systems is key.